Goal:
The purpose
of this lab is to gain experience in the interpretation of spectral signatures
of various earth features and surfaces. Twelve spectral signatures (SS) will be
collected from the Eau Claire region using the polygon function in Erdas Imagine
2013. Once this is done the SS will be graphed the Signature Editor tool in
Erdas.
Methods:
The polygon
tool in Erdas imagine was used to spectrally isolate twelve regions around the
Chippewa Valley, shown as a whole in figure 1, as such:
1)
Standing Water: This SS was taken from Lake Wissota
near Eau Claire (EC), Wisconsin. Care was taken to retrieve the SS from the
center of the lake to ensure the water was still.
2)
Moving Water: This SS was retrieved from a known
high-velocity region of the Chippewa River; just west of the UWEC campus.
3)
Vegetation: SS for forest vegetation was taken
near Knapp, Wisconsin, where numerous forested areas are known to exist.
4)
Riparian Vegetation: SS for riparian vegetation was taken
along the Chippewa River, just north of Lake Wissota.
5)
Crops: SS for cropland was taken just west
of the area where the Lake WIssota standing water SS was taken.
6)
Urban Grass: The SS for this surface was taken
from a golf-course just to the west of Altoona, WI.
7)
Dry Soil (uncultivated): This SS was taken from an open,
uncultivated field near Knapp, WI.
8)
Moist Soil: SS for moist soil was taken near
Knapp, WI as well. However, the location of this particular field was in a
valley, where moisture retention would likely be higher due to runoff and
shade.
9)
Rock: The SS signature for exposed rock was
taken near Big Falls, WI, where massive units of bare bedrock line the Eau
Claire River.
10) Asphalt Highway: The SS for this location was taken on
a paved road in the city of EC.
11) Airport Runway:
This SS was taken at the regional airport in Menomonie, WI.
12) Concrete Surface (parking lot): A SS was taken of the concrete Sam’s Club parking lot in the
city of EC.
Figures 2
through 13 show the graphical representations of the SS that were collected
above. Also, table 1 shows the high and low bands for each SS obtained above.
Table 1.
Overview of the Chippewa Valley
region where SS were taken for this lab.
Analysis:
Water (figures 2 and 3):
Similar
spectral signatures (SS), in terms of graph distribution and/or overall
reflectance, a mostly seen in features that are comprised of similar materials.
For instance, although standing and moving water, the SS of which are
illustrated respectively in figures 2 and 3, vary in terms of overall
reflectance, their minimum/maximum values on the graphs in figures 1 and 2 are
located at similar bandwidths; in this case bands 4/6 (min.) and 1 (max.). One
reason for the similarities in these SSs as represented on the graphs is that
they both represent water, which generally reflects most EME in the visible
spectrum (bands 1-3, but especially 2). In contrast, EME in other bands is
absorbed by water, resulting in less reflectance and, thus, lower overall
reflectance in these bands.
Vegetation (figures 4, 5, 6, and 7):
Similarly, the SSs represented by various
vegetation should exhibit graphic similarities to one another as well. This is
exactly the case for SS collected from forest, riparian, and crop vegetation,
whose graphs are represented in figures 4, 5, and 6, respectively. In fact, the
SS values, in addition to the behavior of their graphs, for both forest and
riparian vegetation were nearly identical. This could have arisen from the fact
that the data for both features was collected in similar locations. For
instance, one would expect that riparian vegetation would have plenty of water
and would be generally healthy.
Since SS data for the forest vegetation was
collected near Knapp, WI, a hilly area with many valleys, it would not be
surprising that data taken here would exhibit similar qualities. This is so
because moisture would be less likely to evaporate from valleys than from more
open areas. So, assuming a positive correlation exists between a plant’s access
to water and its overall health, it would not be surprising that healthy
vegetation, even from two separate locations, would have similar SSs; both in
terms of graph values and behavior.
Crop vegetation, figure5, had a higher
maximum mean SS than either riparian or forest vegetation, about 150 versus
about 130; all in NIR band 4. This overall greater reflectance exhibited by the
SS of cropland compared to the SSs exhibited by riparian and forest vegetation
could be due to the fact that light hitting cropland will be diffused less so
than trees that inhabit forest and riparian environments. Also, cropland would likely
be healthier overall because human intervention such as fertilization,
regulated watering, and genetic modifications that are implemented by seed
companies in order to ensure hardier crops.
However, one type of vegetation did differ
from the three mentioned above: urban grass. SS data for this feature was taken
from a golf course near Altoona, WI (figure 7). While most of the graphic
information for the SS of this feature was similar to that of riparian, forest,
and crop vegetation, the SS of urban grass exhibited higher reflectance in both
the band 1 and band 5, likely due to the fact that golf course grass would
contain a lot more water compared to the types of vegetation mentioned
previously.
Uncultivated Soil (figures 8 and 9):
While the
spectral signature of both moist and dry soil exhibited a similar pattern on
each graph, figure 8 shows that the overall reflectance of dry soil was the
greater across all bands when compared to the reflectance of moist soil (figure
9). This is because the moisture contained within the soil will absorb EME more
readily than dry soil.
One note on these surfaces, however, is that
the actual moisture content of the soil was merely speculated. For instance,
the data for moist soil was collected from an uncultivated field in a valley.
The assumption being that moisture content of soil would be higher in a valley
setting due to factors such as less wind, less direct sun, and more forest
vegetation, as well as topography that may channel runoff to such a location. In
contrast, the data for dry soil was collected from a flat area with few trees
to offer protection from sun and wind that could dry out the soil. However, the
actual soil content of this location is unknown.
Rock (figure 10):
Of all the hard-surface SSs listed in this
lab (i.e. asphalt, rock, and concrete), bare rock (figure 10) had the least
reflectance and graphical representation of the data that was least like the
other three. One reason for this is that the location of this data was on the
Eau Claire River (ECR) near Big Falls in Wisconsin. The bare rock in this area
is likely too small for the spatial resolution of the image (30m). Because of
this the spectral data of the water flowing through this location was likely
averaged into the overall brightness value of the area. Other factors that may
contribute to the high absorption of EME in this area is that the rocks
themselves are dark in color and shadows on them may contribute to higher
absorbance of EME in this area.
Asphalt, and Concrete (figures 11, 12, and 13):
The SS of asphalt on a road surface (figure 11)
had the least amount of reflectance compared to those of a runway and a
concrete parking lot. One reason for this is that dark colored asphalt will
absorb more EME due to its dark color compared to lighter colored asphalt or
concrete.
Figures 12 and 13 (runway and concrete,
respectively) have very similar spectral signatures in terms of the way their
graphs are patterned; at least compared to the SS of the asphalt surface shown
in figure 11. This is likely due to the fact that these surfaces are much
lighter, in terms of color, compared to asphalt and thus reflect more EME.
However, the overall reflectance of the concrete Sam’s Club parking lot is
greater than that of the runway.
One reason for the similarities between the
SS of the surface features at Sam’s Club and the Menomonie airport runway may
be that they are both constructed of similar materials: concrete. However,
differences in the spectral signatures Sam’s Club and the runway may be due to
the fact that as airplanes land they leave skid marks from their rubber tires.
Because of this, the overall reflectance between these two surfaces is
different. With the more lightly colored parking lot reflecting more EME than
the runway.
Figure 2
Spectral signature of water in Lake Wissota.
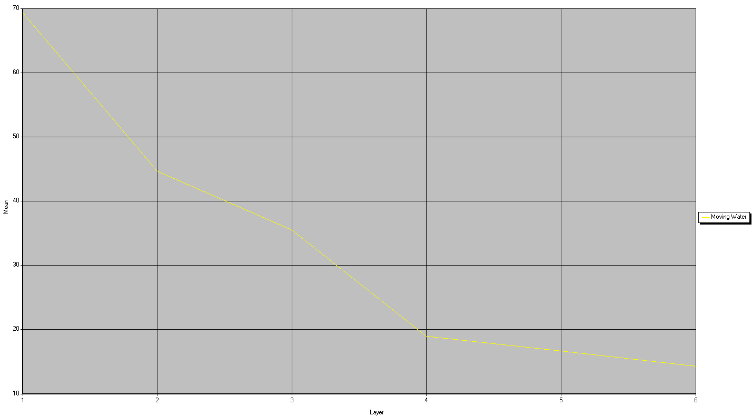
Spectral signature of forest
vegetation near Knapp, Wi.
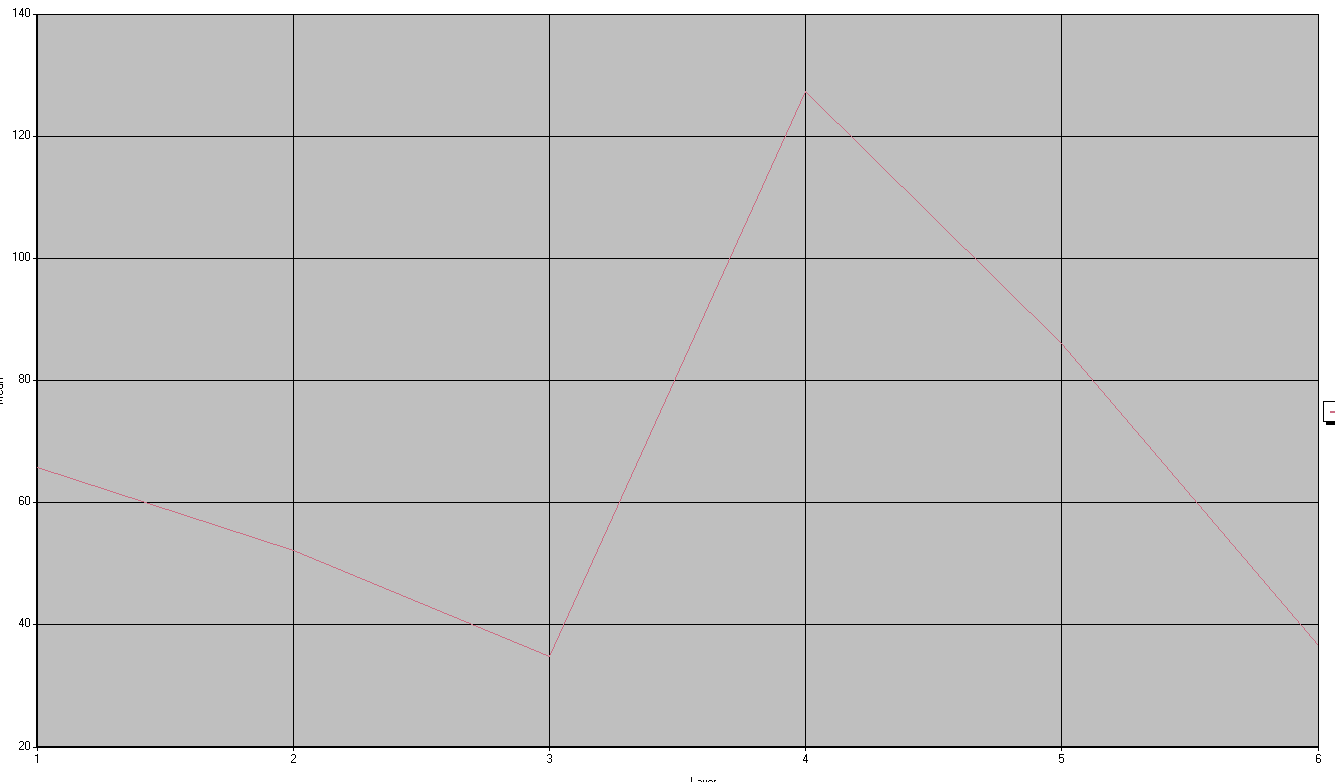
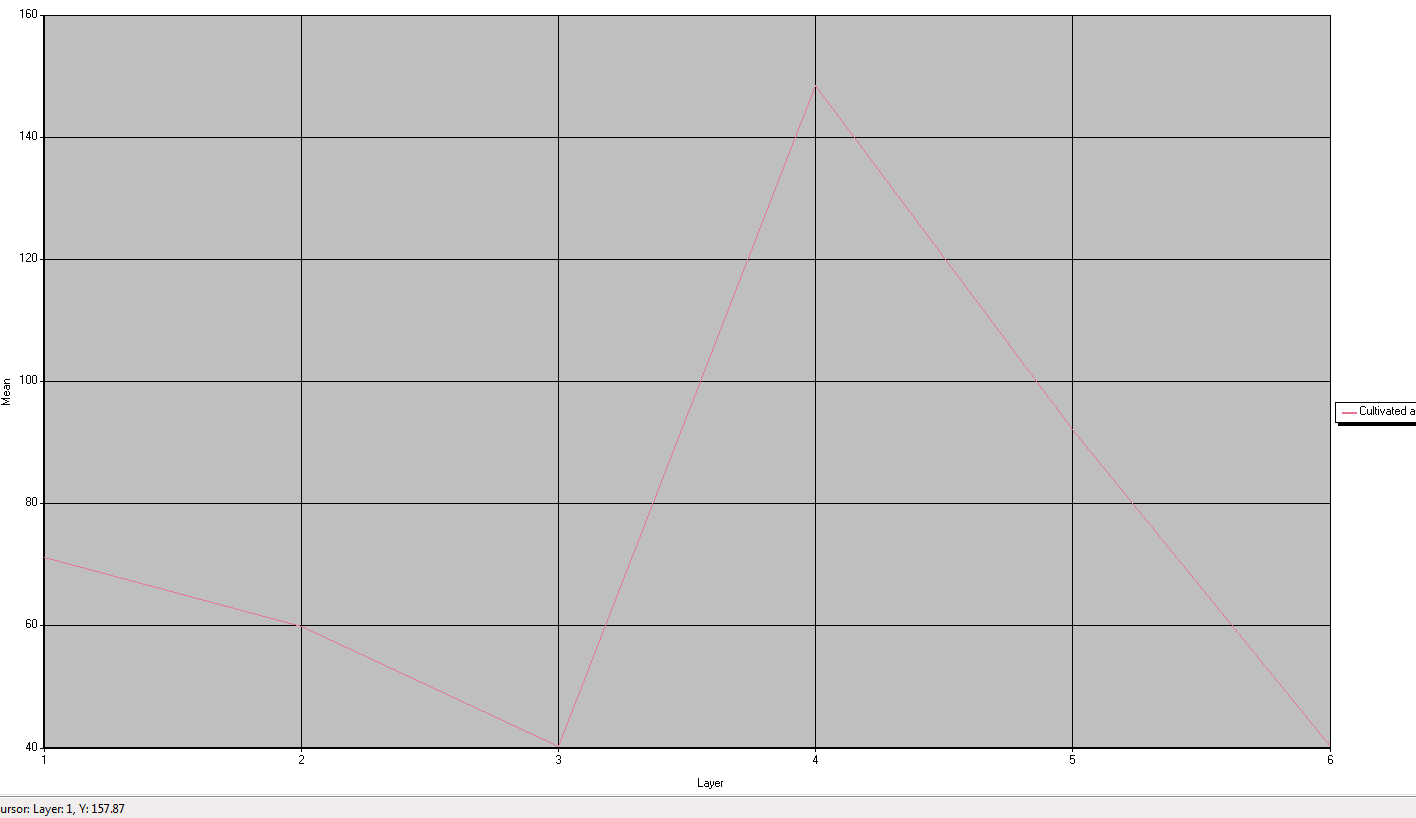
Spectral signature of dry,
uncultivated, open field near Knapp, Wi.
Spectral signature of moist soil in. Uncultivated field in a valley near Knapp, Wi.
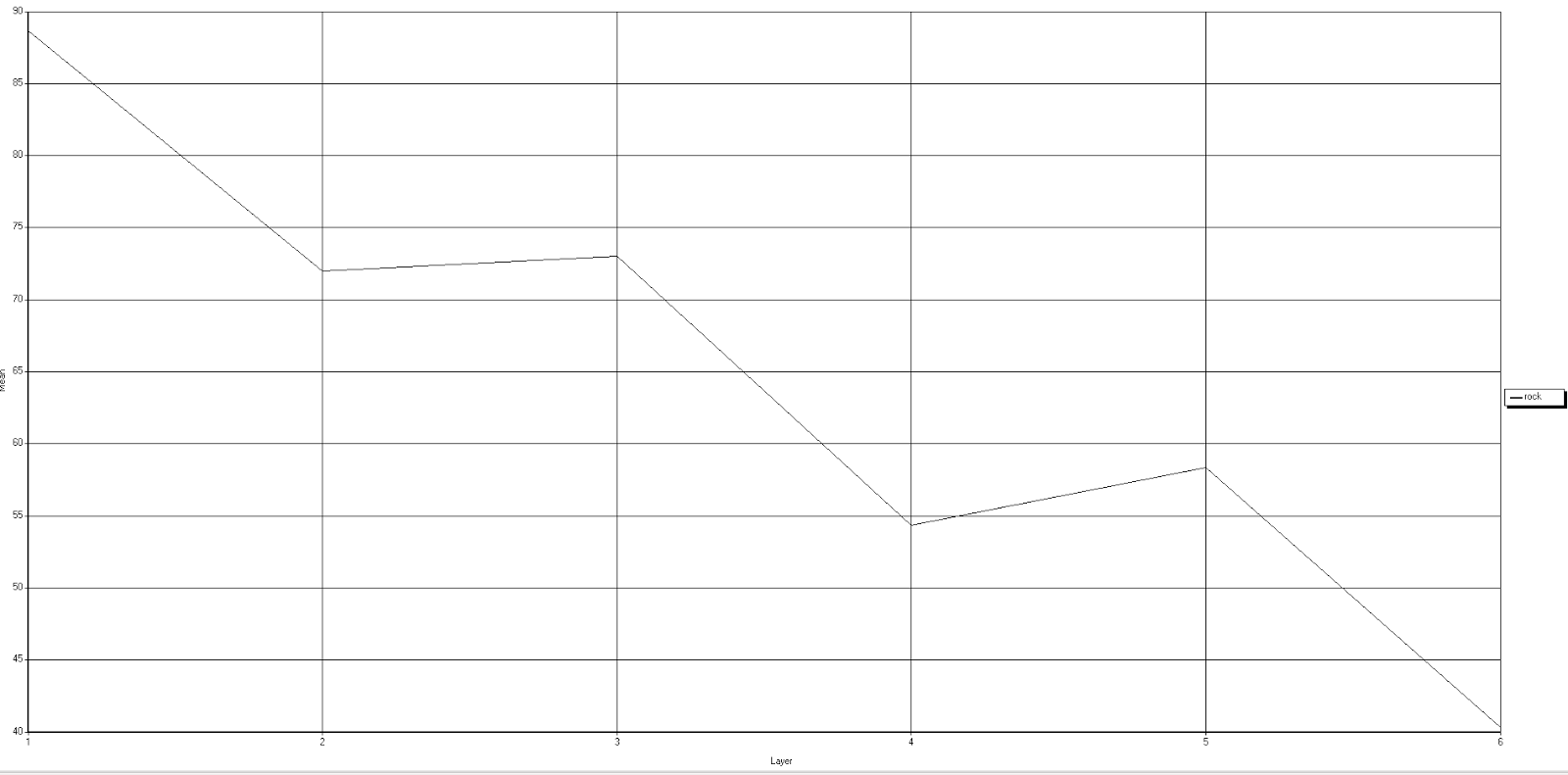
Spectral signature of asphalt on an Eau Claire road.
Spectral signature of an airport
runway (Menomonie, Wi).